GenAI Models: Content Generation with AI
GenAI models have ushered in a new era of content generation through AI (Artificial Intelligence) and are redefining what is possible across a variety of fields. By harnessing the power of GenAI models, businesses have unlocked unforeseen opportunities and pushed the boundaries of creativity and innovation.
In this article, we will introduce the different types of generative AI models and explore their unique features and applications, aiming to develop a better understanding of the mechanisms behind AI-generated content.

What types of GenAI models exist for Generative AI?
- Generative Adversarial Networks (GANs): GANs are a significant advancement in generative AI. They consist of two main components: a generator and a discriminator. The generator’s role is to produce new content, such as images or text, based on certain patterns it has learned from training data. The discriminator, on the other hand, acts as a judge, distinguishing between real and generated samples. Through a continuous feedback loop, the generator learns to improve its content generation based on the discriminator’s feedback. GANs are known for their ability to quickly generate high-quality content, although they may have limitations in terms of sample diversity. This makes GANs particularly well-suited for generating specific types of data within a specific domain.
- Variational Autoencoders (VAEs): VAEs are a type of generative AI model that includes two essential components: an encoder and a decoder. The encoder is responsible for compressing input data into a condensed representation called a latent space. The decoder, on the other hand, takes this latent representation and reconstructs it to generate new data. VAEs have the ability to generate novel data by sampling from the latent space. While VAEs can generate outputs faster than diffusion models, they may not capture intricate details as effectively. Nonetheless, VAEs present a unique approach to content generation and find applications across various fields.
- Diffusion Models: Diffusion models, also referred to as denoising diffusion probabilistic models (DDPMs), have become increasingly popular in the realm of image generation. These models have shown superior performance compared to previous methods like GANs and are adept at producing high-quality outputs. The process employed by diffusion models involves two stages: forward diffusion and reverse diffusion. During forward diffusion, random noise is added to the training data, creating a diverse range of samples. In the reverse diffusion stage, the models reconstruct data samples by undoing the added noise. Diffusion models can generate various types of content, such as realistic images, artwork, or visual designs, with impressive detail and quality. While diffusion models offer flexibility and high-quality results, it’s worth noting that the reverse sampling process can be time-consuming.
- Transformer-based Models: Transformer-based models have revolutionized natural language processing. These models also referred to as Large Language Models (LLMs), process sequential input data in a non-sequential manner using self-attention and positional encoding mechanisms. By effectively analyzing the relationships between different parts of the input, transformer networks can decipher and predict patterns within text data. This makes them highly effective for text-based generative AI applications, such as generating coherent and contextually relevant content for various purposes like news articles, creative writing, and code snippets. Transformer-based models have significantly advanced the field of text generation in AI.
From GANs and VAEs to diffusion models and transformer-based models, each type of GenAI model brings its own strengths and applications. As these models continue to evolve, their impact on various industries and creative projects will undoubtedly grow, shaping the future of AI-driven content generation.
If you’re interested in exploring the potential of GenAI models and leveraging their capabilities for your business, our team at theBlue.ai is here to develop custom generative AI solutions tailored to your specific requirements. Feel free to contact us for more information and take the first step towards scalable and impactful AI solutions.
Application Areas for Generative AI
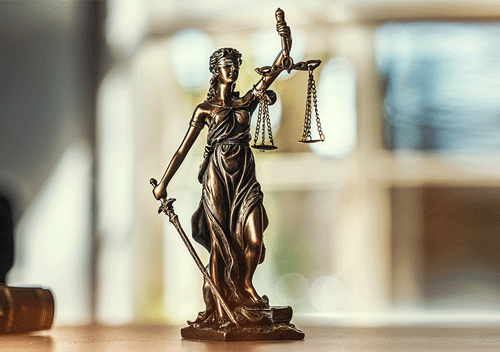
Generative AI in the Legal Industry: Generative AI offers diverse opportunities for optimizing business processes and supporting lawyers. With the right GenAI models and a fitting solution for your business, it becomes possible to automatically generate contracts and create important documents. AI enables the potential for efficient examination and analysis of documents. A virtual assistant has the potential to provide intelligent advice and summarize information. This opens up the possibility of increasing efficiency, saving time, and providing support in legal work. Learn more.

Generative AI in Education: Advanced AI technologies enhance learning in the education sector. It becomes possible to automatically generate suitable learning content, generate quiz questions, and create summaries. Papers can be automatically corrected and improved. Interactive learning activities have the potential to foster student engagement. Generative AI opens up the possibility of personalized and effective learning. Learn more.
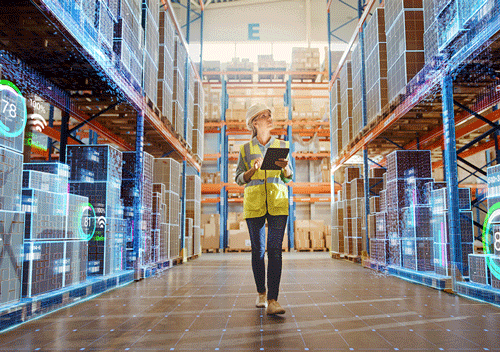
Generative AI in Retail: Generative AI solutions have the potential to fundamentally transform the retail industry by addressing specific challenges and supporting various use cases. In customer service, virtual assistants based on GenAI models can potentially handle customer inquiries efficiently, creating an improved customer experience. The automatic generation of consistent product descriptions could ensure a unified presentation of products. Additionally, GenAI models enable the potential for creating personalized product images, individualizing the shopping experience, and appealing to customers. With these solutions, retailers have the potential to optimize their business processes and provide a tailored shopping experience to their customers. Learn more.

Generative AI in Finance: The application of generative AI in the finance industry opens up opportunities to optimize business processes and enhance the customer experience. GenAI models can facilitate the efficient processing of financial documents. A virtual generative AI assistant could provide valuable support. Tailored financial reports can be generated. Fraud detection can be improved. Clear rejection statements for bank customers can be automatically generated. Visual reports and graphics can offer an illustrative overview. Generative AI has the potential to strengthen the finance sector through efficiency and customer satisfaction. Learn more.
Introduction to using Generative AI
To take advantage of the benefits of Generative AI, you can start integrating the technology into your business model. The following approach has proven successful:
- Identify a suitable use case and collaborate with AI specialists to evaluate the use of Generative AI.
- Create a proof of concept to test the effectiveness of the technology.
- Upon successful completion of the proof of concept, models are created and configured to scale the use of the technology in various use cases.

Custom Generative AI Solutions for Your Business
At theBlue.ai, we specialize in developing customized Generative AI solutions using cutting-edge GenAI models for businesses across various industries. With our expertise and experience, we work closely with you to create tailored solutions that harness the power of GenAI models and meet your specific needs. Whether you’re looking to automate content generation, enhance data analysis, or optimize decision-making processes, our generative AI services can help you achieve your goals.
Discover the possibilities of Generative AI. Contact us today to learn more about our generative AI services powered by GenAI models and start your journey toward scalable and impactful AI solutions.